[SPONSORED CONTENT] In this interview conducted on behalf of Dell Technologies, insideHPC spoke with Dr. Paul Calleja, director of Research Computing Services at the University of Cambridge, about the Wilkes3 supercomputer, currently ranked no. 4 on the Green500 list of the world’ most energy efficient supercomputers.
Dr. Calleja discusses how he and his team developed an low-power strategy for the 80-node Wilkes3 system by the adoption of GPUs and lower clock speeds balanced against high throughput. He also explains how the Dell PowerEdge XE8545 -based cluster, which utilizes AMD EPYC CPUs and the Mellanox InfiniBand interconnect, fits within the university’s larger, heterogeneous Cumulus system, comprised of 2,500 Intel x86 servers.
It’s all part of Dr. Calleja’s efforts to reorganize the university’s HPC capabilities, the result of which is that Cambridge now boasts the fastest academic supercomputer in the UK, according to the university.
Doug Black: Today on behalf of Dell Technologies, we’re talking with Paul Calleja, he is director of Cambridge University’s Research Computing Services, where he and his team developed the Dell-based Wilkes3 supercomputing cluster. Paul, welcome.
Dr. Paul Calleja: Thank you.
Black: So we know the Wilkes3 system attained the number four spot on the Green500 list of the most energy efficient supercomputers. Tell us about the focus on energy efficiency at Cambridge.
Dr. Calleja: Yeah, that’s a good question, Doug. So green issues are really high on the university’s agenda. Currently, the university has set a very aggressive carbon zero roadmap where it wants to really drive down its energy use across the whole estate. Of course, supercomputing — when you look at the university’s top energy users — supercomputing is up in the top five, we suck more energy than a large department.
So there’s a lot of focus on, what can we do to lower that energy cost, lower the energy consumption. And the UK Funding Council, the science funding council, now also have a strong focus on reducing the environmental impact of UK science and engineering. And again, supercomputing comes high on that list. So there’s a general trend, both within the university and science funding activities in the UK, to drive down energy consumption where possible.
Black: Okay, great. Please share some details about the Wilkes3, how many servers it comprises, the processors you’re utilizing and other characteristics.
Dr. Calleja: When you’re looking to drive energy consumption down in HPC, you really look towards GPU computing — GPU computing is much more energy efficient than traditional x86 based systems, so we looked to build out a GPU system. We’ve been building large-scale GPU systems at Cambridge for many years, five, six or seven years. And this is our third machine, hence the name Wilkes3.
We use NVIDIA GPUs within a Dell server platform. The x86 CPUs in that platform are AMD, which at that time last year were the only PCIe Gen 4 system, so we need PCIe Gen 4 for the bandwidth. The GPUs are Nvidia’s A100 GPUs, that’s with the four-way NVLink. So there are four A100 GPUs linked by NVLink, they sit inside the Dell PowerEdge server, and in that server we have two Mellanox (InfiniBand HDR 200 (Gb) network cards to get that bandwidth out. And so that’s one unit — two AMD CPUs, four A100 GPUs, two Mellanox HDR 200s, that’s one unit. We have 80 of those units in total, so that’s 320 GPUs. And that’s 4.5 to 5 petaFLOPS of computational power.
In order to get that energy consumption right down, we actually customized the platform by turning down the clock speed of that GPU. You can really make very large energy savings on GPUs by turning down the clock speed. So we’ve found a reduction in clock speed from 1355 megahertz, which is the default, down to 1095 reduces the LINPACK performance by just 10 or 11 percent, but you save around 35 to 40 percent power. So there’s really large energy savings with quite a small performance savings from turning down that clock speed. And that’s how we got the number four position in the Green500.
Black: So that’s kind of a favorable tur down that you see occurring….
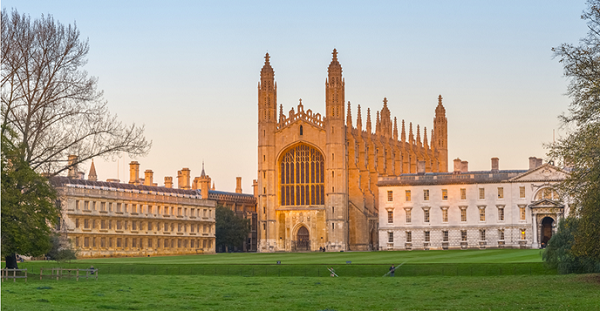
University of Cambridge
Dr. Calleja: In all these things, actually, the Green500 is a little bit misleading because it just measures the power consumed during the job. But actually, what you should really be measuring is the energy-to-solution for that job. Because of course, if you turn down the clock speed and turn down the power you increase the time taken. So just a measure of the power during the job doesn’t tell you what you want to know. What you want to know is does the power reduction, when you look at the whole length of the job, have you consumed less power? That’s what we really looked to do. As you say, power time to solution goes up.
Black: I guess my point is that as you turn down clock speed there’s not a corresponding loss of power, in fact it’s actually favorable,
Dr. Calleja: It’s favorable because of course — this is all well-known physics, right — so the energy is related to the clock speed squared. Time is related to this linear clock speed. So you save more power than the time increase. So this squared relationship with clock speed is really quite useful. The length of time it takes is less than the amount of energy you save because of this squared relationship with clock speed.
Black: Okay, now is Wilkes3 part of the Cambridge’s Cumulus system?
Dr. Calleja: Yes, it is. The Cumulus system is a much larger, heterogeneous system that we have in total. So we have around 2,500 Intel x86 servers connected to the GPU servers all within the same Mellanox network. We find now that workflows exist well in a heterogeneous architecture, so you can have converged workloads or simulation and AI and data analytics. So we like to have an environment where those jobs can coexist. And you have different architectures of compute for different types of workloads.
Another facet of the Cumulus system is that we run this within an open stack cloud-native environment. So the whole of that Infrastructure, the whole 10 petaFLOPS, is presented via an open stack cloud-native interface. And that really increases the flexibility of the system so we can let different stakeholder groups have their own customized tenancies within that cloud-native environment. And that’s a very important part of the way we provide services to our users.
Black: Okay, so now tell us how Wilkes3 fits in with the Open Exascale Lab.
Dr. Calleja: The Open Exascale Lab is an exciting new initiative funded by Dell and Intel. It’s quite a large investment into Cambridge, to hire around 20 engineers to look at the whole range of activities that come under this totem of exascale. Exascale is a good totem, it’s a signpost, the next “almost here” pinnacle in computing in terms of size. And of course, to deliver exascale practically, especially outside of the few beachhead installments, once you want to get exascale out to the masses, it has to become a lot more tractable. And the problems of exascale, of course, are the size of the systems needed, power, the usability – there’s a whole host of tactical problems that need to be addressed for exascale to be democratized.
And so they’re intelligent enough to look at a whole range of these pinch points, in terms of making exascale technology usable by the masses. And of course, one of those pinch points, as I said, is the power. So energy consumption of exascale systems is an issue, and we’re looking at that with a number of technologies.
Of course, just turning the clock speed that I mentioned is a very blunt tool. And within the Open Exascale Lab, one of the things we’re looking at is using Intel … (tools) to monitor in real time within the system what’s going on in terms of energy consumption and utilization. You can turn the clock speed up and down in real time within the process; core times, so you can turn some cores down, allow other cores to go up. So there’s much more fine-grained ways of changing the clock speed of particular elements of your infrastructure in response to what’s going on. And that’s one of the main activities in the lab, focused on energy consumption at the moment.
Black: That’s fascinating. I would assume that’s workload dependent?
Dr. Calleja: It is workload dependent., and it’s also timing within the workload dependent because of course a particular application at different times in its execution is doing different things at different times. And (Intel) monitors that and can turn down the frequency of calls that are not being used. And you can use that frequency saving in different ways. You can yank it and do nothing with it and save the energy, or you can allow the energy saving to turn other processors, other cores, up, (so) you can have both ends of the stick, you can save power and make your application go faster at the same time. So this is actually quite interesting. We’re going to be writing up several papers with Intel early to mid-year.
Black: We’d love to hear more about that as that comes together. Tell us about some of the work your clusters are doing, some of the work the system support.
Dr. Calleja: Cumulus, that 10 petaFLOPS heterogeneous system, is a UK national HPC resourse, so we serve communities at the university and we also serve communities outside of Cambridge. One of the facets of the system is that it’s really cross domain, so we have users from a broad cross section of the scientific and engineering community – obviously, the usual suspects in physics, chemistry, engineering, observational astronomy, those kind of traditional HPC users.
But I think more interestingly, we have had a recent focus on how do we take HPC resources, AI resources, data analytics resources, into clinical medicine? How do we get these technologies usable within the clinical environment? That was actually one of the drivers for our open stack, middleware environment, to create sandbox securities areas where we could hold patient data in a secure way but let that data be computed on. We normally have a clinical store, they have secure storage for holding data, but they can’t do anything with it. You can’t expose that data then to a high performance computing system, because the security models are too open.
So we created an open stack environment that allows us to build dynamic, secure tenancies of clinical data. Now we see how resources have been used in many areas of clinical medicine, from brain image analysis to cancer genomics. Recently, of course, there’s been an awful lot of COVID work, Cambridge supports a lot of the UK government’s modeling activities for COVID, we do a lot of COVID workup with the hospitals in profiling patient data and analytics of COVID. We do a lot of modeling work with people trying to chart this modeling of COVID. Really, COVID is just a good segment of the work that we’ve been doing for years, and COVID puts a big spotlight on it. And of course, the urgency of that work is important. So you have to have workflow environments if you push those jobs up through the system quickly, and that’s where scale really comes in useful to get that workload done urgently.
Black: My own opinion is supercomputing, HPC, is an unsung hero of COVID-19.
Dr. Calleja: Yes, there’s been a lot of great work on that, especially in the States. I do a lot of work with the guys at TACC (the Texas Advanced Computing Center), and the work we’ve done over there, that’s a real showcase of what can be done at scale. Of course, the TACC systems are another order of magnitude up from our size of systems. And you can see that the scale really matters when you need to do things quickly and you need to do things urgently.
Black: Yes, great stuff. We’ve spent a little time with Paul Calleja at Cambridge University. On behalf of Dell Technologies, thanks so much for your time today
Dr. Calleja: Thank you.